Navigating the Data Landscape: Insights and Challenges in the AI Era
Here’s a look at the top takeaways from our recent Future Frontiers event, as told through 5 of our favorite quotes.
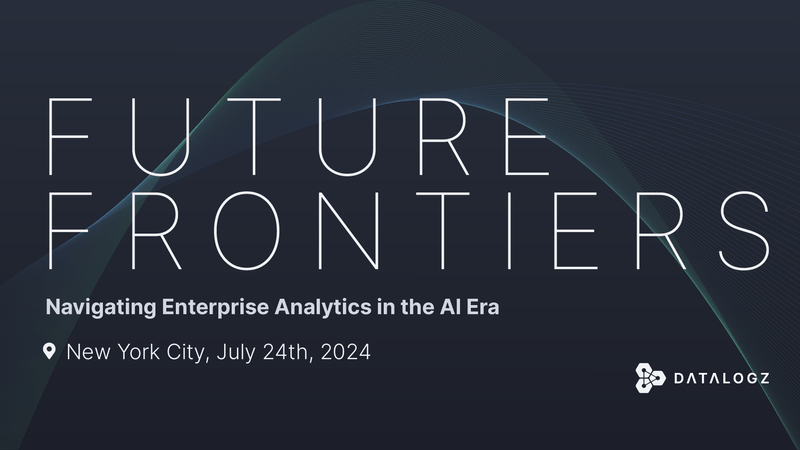
Preparing to enter the AI era of the near future doesn’t mean we get to leave the data challenges of the present behind.
For enterprises, it’s more clear than ever that harnessing the power of data and analytics to unlock efficiency and create new value is critical to driving results and gaining a competitive advantage. That is only underscored by the expanding number of business intelligence platforms at leaders’ disposal, and the increasing demand for Generative AI from executives and board members. Data is trending, and the coming shift is poised to reshape C-suites, budget priorities, org charts, and talent.
But as automation and analysis tools move from the promise of innovation to the practicalities of scale, business and government leaders must confront challenges with BI sprawl, data governance, and advancing data-driven culture that is core to effective data management. The increasing speed and volume of data accelerates these struggles, meaning there is more need for guardrails to create trust and ensure effectiveness, not less.
What we are really talking about is another wave of transformation, and it is one that will give data leaders an increasingly influential role within organizations. This means they must think like business leaders, just as much as technical leaders. It’s not enough to introduce new tools and ensure they are working. Leaders have to ensure these tools work together, work for people, and serve the goals of the business.
These were among the takeaways for Future Frontiers: Navigating Enterprise Analytics in the AI Era, a recent panel discussion hosted by Datalogz that gathered over 50 CDOs, CISOs, and Heads of Data/BI/Analytics from Fortune 1000 companies.
The panel featured remarks from two data leaders with decades of experience: Guy Lehman, a data and transformation leader who served in top roles at Walmart and Honeywell, and Xingchung Liu, PhD, Chief Commercial Analytics Officer of Pfizer. Datalogz CEO Logan Havern served as the moderator of the panel.
Here’s a look at the top takeaways from the event, as told through 5 of our favorite quotes:
‘Leverage Data to Make an Impact on People’
From the opening introductions, the panel showcased how a career in data and analytics presents a diverse set of opportunities that can yield notable success and massive impact.
“I love this industry, I love this space,” said Lehman. “The concept of data analytics is near and dear to my heart.”
Over the last three decades, Lehman and Liu have applied data expertise to grow startups from the ground up, and power digital transformation at the world’s most influential enterprises.
Lehman first saw the power of data and analytics while serving as a global data leader at Dun & Bradstreet, before moving to startups in the credit and healthcare spaces, respectively. He later went on to pioneering enterprise leadership roles at Honeywell and Walmart, developing strategies and building teams to help these leading corporations use data to unlock new layers of value. This experience has made him a sought-after advisor to companies on a data transformation journey.
Liu put training as a data scientist to work through a decade-long run with three startups, each of which went on to a successful exit. He then served in top data and analytics roles at The Home Depot and Macy’s, powering both retailers to adopt a data and AI-driven culture. He now serves as the Chief Commercial Analytics Officer at Pfizer, applying the customer-driven mindset that has powered the consumer economy at a top pharmaceutical manufacturer.
For these leaders, time spent in both the startup and enterprise worlds has provided plenty of perspective. In the startup world, Liu said the focus is on moving fast and solution development. In the corporate world, the focus is on integration, adoption, and scaling solutions.
Throughout these careers, growth was driven not only by the successful implementation of technology but also by what it achieves. No matter where the work is taking place, Liu said he maintains a focus on, “how we can leverage data analytics to make an impact on people.”
‘Think About Your Talent of the Future’
Bring up data and analytics in 2024, and it’s not long before the conversation winds around to the topic of Generative AI. Thanks to rapid technical development and its democratization via services such as ChatGPT and Microsoft Co-Pilot, many business leaders have doubled down on the promise that capabilities leveraging existing data to create new content will reshape the digital economy, and how we work.
As compelling as the recent advances may be, Lehman offered a reminder that AI has been around for a long time, dating back to the 1950s. It has been a sought-after tool by enterprises for nearly just as long. In fact, many of the use cases that helped propel AI forward originated in the business world. After all, it offered proof points. The value of using data and analytics to drive decision-making and automate manual tasks could be tied to real business outcomes. Over the years, these tools were able to take on increasingly complex workloads with more efficiency as data and compute power became more plentiful, and machine learning techniques became increasingly proficient. The recent explosion of Generative AI has not only familiarized the general public with AI as a concept, it has shifted the prism through which we look at AI from analysis to creation. AI has the promise to become a tool that you interact with to build something new, complementing existing tools that can be programmed to dig deep into data and analyze the results. Increasingly, the power to leverage large-scale data analytics is in everyone’s hands. This push has been accelerating for years, but the popularization of generative AI stands to make these tools increasingly in demand.
“The concept of leveraging a capability like this is really important at a company, not only to build out new products and services but also to think about your talent of the future,” Lehman said.
Increasingly, students are using Generative AI, and they will be expecting to use the associated tools when they enter the workforce. When adopting the technology, business leaders should consider how it can be deployed to gain a competitive advantage and shape the workforce in the years and generations to come.
It will change both how we work, as well as our work product.
‘Data is the Differentiator’
Practical applications of Generative AI are beginning to proliferate. Companies are already leveraging AI products to assist with office tasks, generate marketing content, drive business decisions, and realize new avenues of profitability. The potential to create new value is vast. Walmart harnessed its data to create new analytics products for suppliers and built a new B2B revenue line in the process.
But at the same time, the arrival of Generative AI in the American corporation is not as fast as predicted. Unrelenting attention to AI has created a mismatch between expectations and reality at some companies. While products are being developed every day, leaders are running into challenges related to governance, security, maturity, reliability, and configuration of their data.
These are the same challenges that companies face in all facets of data, from lakes and warehouses to the sprawl of reports created by the growing number of business intelligence platforms.
Instead of alleviating these struggles, Generative AI will only accelerate the difficulties of managing data at scale. Techniques that rely on foundational components such as Large Language Models allow more data to be analyzed at a faster pace, and it is more important than ever that it is reliable. So it will be more important than ever to confront issues related to duplication and unused data while putting controls in place around governance and access. At the same time, rolling out products at scale with still-developing technology presents risk. There have been high-profile challenges over bad advice from a chatbot, inaccurate orders on AI drive-thrus, and legal challenges to ownership of the data used to train the large language models that undergird Generative AI.
Technologists are moving this innovation from the possible to the real. But, just as they did in previous generations, businesses will determine how that technology is applied in the world. In sophisticated industries, that doesn’t happen overnight. As they see the first demos of new products promising productivity gains, board members and executives are excited to roll them out. However, there is a lot to consider when rolling out data-driven technologies across an enterprise, from processes and workflows to culture and security.
“The pipelines at most companies I speak to are full of these products ready to launch, Lehman said, “but there’s a lot to rolling this out across the enterprise.” Companies are “reticent,” he said, due in part to “murky rules around governance and access rights to the data.”
At industry conferences, the tenor of sideline and happy hour conversations has also changed. On the Gartner Hype Cycle, a year ago we were near the Peak of Inflated Expectations, whereas now we’re heading toward the Trough of Disillusionment. But there’s a reason it’s a cycle. Even if there is less froth, leaders can now enter a quieter period of building no less committed to staying the course, and focus instead on showing results.
“The next six months is a great opportunity for cooling down, rational thinking, and getting focused on what we can do next,” Lehman said.
Early adopters who have been laying strategic and technical groundwork for years are in a prime position to leap ahead, but there is still much for organizations to learn over the next two years.
The data itself must be reviewed to ensure it is up-to-date, accurate, and non-duplicative. Risk must be assessed. Enterprises must get their data house in order. After all, the true value lies in the proprietary information and statistics that make a business unique.
“If we all use the same AI technology, at the end of the day there is no difference, so what is the differentiator? Data is the differentiator. That’s where you start first,” Liu said.
‘The Hard Part is About People’
Whether Generative AI becomes widely adopted or not, panelists agreed that data capabilities will continue to evolve, and reshape organizations in the process. One impact could be felt in C-level roles. More organizations have adopted the role of Chief Data Officer and Chief Data and Analytics Officer. Going forward, Chief Transformation Officer may become the norm, reflecting once again that success isn’t only determined by technology prowess.
“The hard part to change is not about technology, the hard part is about people,” Liu said. “That’s what transformation is more focused on — I’ve got to change people’s behavior first, then we can embrace the power of technology and data. Without recognizing that, I think companies will continue to struggle.”
For Lehman, time spent on the road over the years talking to colleagues about data transformation mostly wasn’t spent on technical issues.
“The main part of my role was communication, alignment, partnership, and getting folks to come with me on the ride as opposed to standing back. Those are the hardest issues, for sure,” he said.
Ultimately, Lehman said, continuous innovation and transformation is an “organizational challenge.” He offered a rubric that leaders can use to determine success.
“What’s your business knowledge and acumen? Is your data AI-ready? Are your AI and analytics in place and working? What’s your organizational psychology around this? You can multiply all of those things together to determine the likelihood of outcome, and if any one of those is 0, your answer is 0,” he said.
‘Data and Analytics are Both Assets,’ Not Commodities
Whatever their title, leaders would be well-served to think like a profit center, rather than a cost center. It’s a shift in mindset. The leader of a profit center aims to understand the business inside and out and position themselves as a partner in its success. They are thoughtful with funding and strategic about the work performed. They measure everything. Don’t mistake — this doesn’t mean that a data leader should be expected to generate profit immediately. Rather, the goal is to show how data and analytics are enablers of a growth curve.
“It doesn’t mean you spend money today and tomorrow you’re going to double it. It’s exponential,” Liu said. “You’ve got to have that patience and commitment, then you’re going to see the return over time.”
In the end, a data leader must be a business leader. They can’t be separate. This puts a priority on effectively managing data.
Data growth has accelerated, and a CEO needs to trust that the information underlying the company is correct. Data is the living and constantly changing representation of your business, and it can’t lie.
“Now the truth is there in all of your systems,” Lehman said. “Companies, because they weren’t controlling this, data has sprawled and created a huge challenge. The idea of tools that can automate that and drive the concept of data analytics being an asset to the company is really critical. You treat an asset very differently than you do a commodity and I think data and analytics are both assets.”